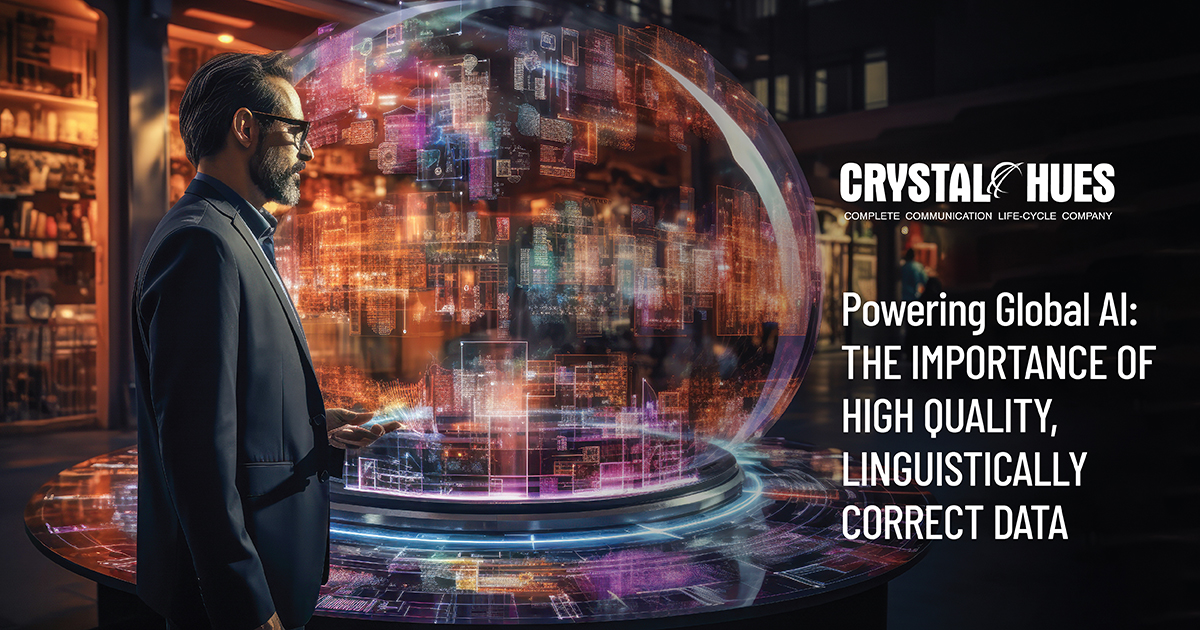
Powering global AI: The importance of high quality, Linguistically correct data
Using Localization Expertise to Deliver Better AI Data Services
AI has transformed industries around the globe and continues to do so. Further, it is changing how we live, work, and interact across the world. From serving multilingual customer support chatbots to conducting global behavior analysis, it may seem that AI has no boundaries. However, every truly effective AI application (with a global remit) must have a key ingredient at its foundation: high-quality, culturally favorable, linguistically true data. AI algorithms, and hence LLMs, only work well if their data is more than correct and reliable, which in turn depends on their familiarity with the characteristics of language and culture.
When it comes to AI, whether it is in the form of a robot, an algorithm-based system, or an LLM, the quality of the data used for training directly dictates the performance of the AI model. The data it was trained on is the foundation upon which the AI systems are developed, no model can ever work without data. Poor quality data, whether it be incomplete, inaccurate, inconsistent, out of date, or culturally insensitive, will lead to unreliable predictions from the AI model, making poor quality decisions in diverse contexts, and often results in AI that subsequently perpetuates harmful linguistic or cultural biases.
High-quality data, particularly in a multilingual AI context, has several characteristics:
- Accuracy
Data must be correct and linguistically precise, with correct grammar, terminology and cultural meanings. Errors in translations or inappropriate culturally toned capabilities will yield skewed outputs and erroneous insight.
- Completeness
Datasets need to represent the range of languages, dialects and regional variations that are applicable to the AI's application. Missing perspectives or low representation of specific linguistic groups could result in biased outputs.
- Consistency
Data must be consistent in meaning and structure/format across different languages and sources. Inconsistent terminology or different associative meanings could make it difficult to identify reliable patterns and meanings by AI models.
- Relevance
The data must be relevant to the particular problem and the specific cultural/linguistic context for which the AI has been specifically designed. Irrelevant data brings noise, whereas culturally irrelevant data brings ineffective or inappropriate outputs.
- Timeliness
Data needs to be reflective of current linguistic usages, culturally influenced usages and the current real-world relevant information sources that apply. Out-of-date information creates erroneous outputs, particularly in dynamic, evolving global markets.
If the quality of data is neglected, particularly the linguistic and cultural aspects of quality, you will end up with highly ineffective AI systems on a global deployment, leading to operational inefficiencies, alienating potential users, tainting or damaging brand reputation, and risking non-compliance with local expectations.
The Human Touch: The Invaluable Role of Linguistic and Subject Matter Experts
Even though AI can process data at scale, human expertise is still valuable when decoding the intricate nature of language and culture in the world today. AI Data service providers utilize a diverse global network of linguists, cultural consultants, and domain-based Subject Matter Experts (SME).
Their contributions are key to AI data annotation in several ways:
Multilingual Data Curation and Annotation
Subject Matter Experts can provide the linguistic and domain expertise necessary for choosing, cleaning, and accurately tagging data in multiple languages. Subject matter experts consider regional dialects, idioms, and contextual considerations in ways that an automated system cannot. These contextual, cultural, and linguistic subtleties are vital for accurate AI outputs in multilingual text classification, sentiment analysis, named entity recognition, etc.
Ensuring Cultural and Contextual Suitability
Cultural consultants work with language experts to ensure the data is maximizing alignment with both localized use cases and specifically targeted locales to ensure the AI is behaving appropriately and effectively when it's deployed in myriad cultural contexts.
Providing Language and Cultural Context
AI models don't build in context. Subject matter experts offer the context necessary to imbue the subtleties of language and culture that are pivotal to developing well-rounded and capable LLMs.
Validating AI Multilingual Models
Subject matter experts test LLMs after training to validate the model's behavior in real-world contexts. They ensure that various multilingual outputs provided by the AI model share performance consistency across languages and regions, such as seen in trend assessment, accuracy, overall relevancy, cultural sensibility, and, ultimately, fairness in factual outcomes.
Dealing with Linguistic & Cultural Bias
To de-bias multilingual datasets, an expert must identify the biases present, and an expert then mitigates those biases, leading to fairer AI performance operating on multilingual datasets for broader populations.
This combination of technology and applied human linguistic skills will lead to trustworthy AI systems that can operate via globally competent AI. Companies with roots in localization provide immediate access to these capabilities.
Working in the language field: Multilingual AI data services' problems
There are significant challenges to developing high-quality data for global AI:
Data Bias
Bias in training data can produce AI systems that unintentionally perpetuate linguistic discrimination (i.e., preferences for dominant languages/dialects) or cultural stereotypes. Identifying and mitigating biases in data requires intentional engagement and cultural knowledge.
Managing complex multilingual data
Managing a large amount of data for many languages, including various scripts and dialects and formats (for example: textual audio and video) requires people with specialized infrastructure and performance.
Data integration and silos
Putting multilingual data together from different global sources with different quality and/or formats is a significant barrier to making holistic conclusions.
Data privacy and security at the global level
This is a major concern in maintaining sensitive data at different global privacy standards (i.e., GDPR, CCPA, etc.).
Addressing these demands in the future involves strong data management strategies, development practices, tools, and most importantly, a global context – something that we have in our localization expertise.
Looking into the future - Trends Reinforced by Localization Expertise
In the rapidly evolving AI data services space, we see trends in areas where localization expertise can provide value:
Emergence of Domain and Locale Specific AI
Training AI on data specific to a niche industry and specific to a region or language elevates the accuracy of the outputs. Companies that provide AI data services can pull together these specific multilingual data sets for these specific domains.
AI Enabled Data Quality Management
AI tools assist with automating data quality checks. However, they do not replace humans in overseeing the linguistic checks to validate accuracy and cultural appropriateness in a multilingual context.
Build Data Readiness for Global AI
Organizations need robust data ecosystems for global AI uses that are well-governed, secure, mitigated against bias, enriched, accurate and high-quality across languages.
Increased Focus on Cross-Cultural Ethics & Governance
Issuing public statements ensuring fairness, transparency and accountability to different linguistic and cultural groups will escalate.
Emergence of Specialized AI Data Services
The growth of services to provide high-quality, linguistically accurate multilingual data collection, annotation, and evaluation, or LLM fine-tuning services, is now emerging and growing rapidly.
Concluding thoughts
Data that is quality data, linguistically and culturally appropriate data, is not just a requirement for successful global AI; it is the building block upon which the future of analogous intelligent systems in the world at large will rest. By establishing data quality as a priority, building on the irreplaceable role of linguistic and subject matter experts, and beginning to think critically about the bias and complexity in multilingual data, we can realize the full potential of AI.